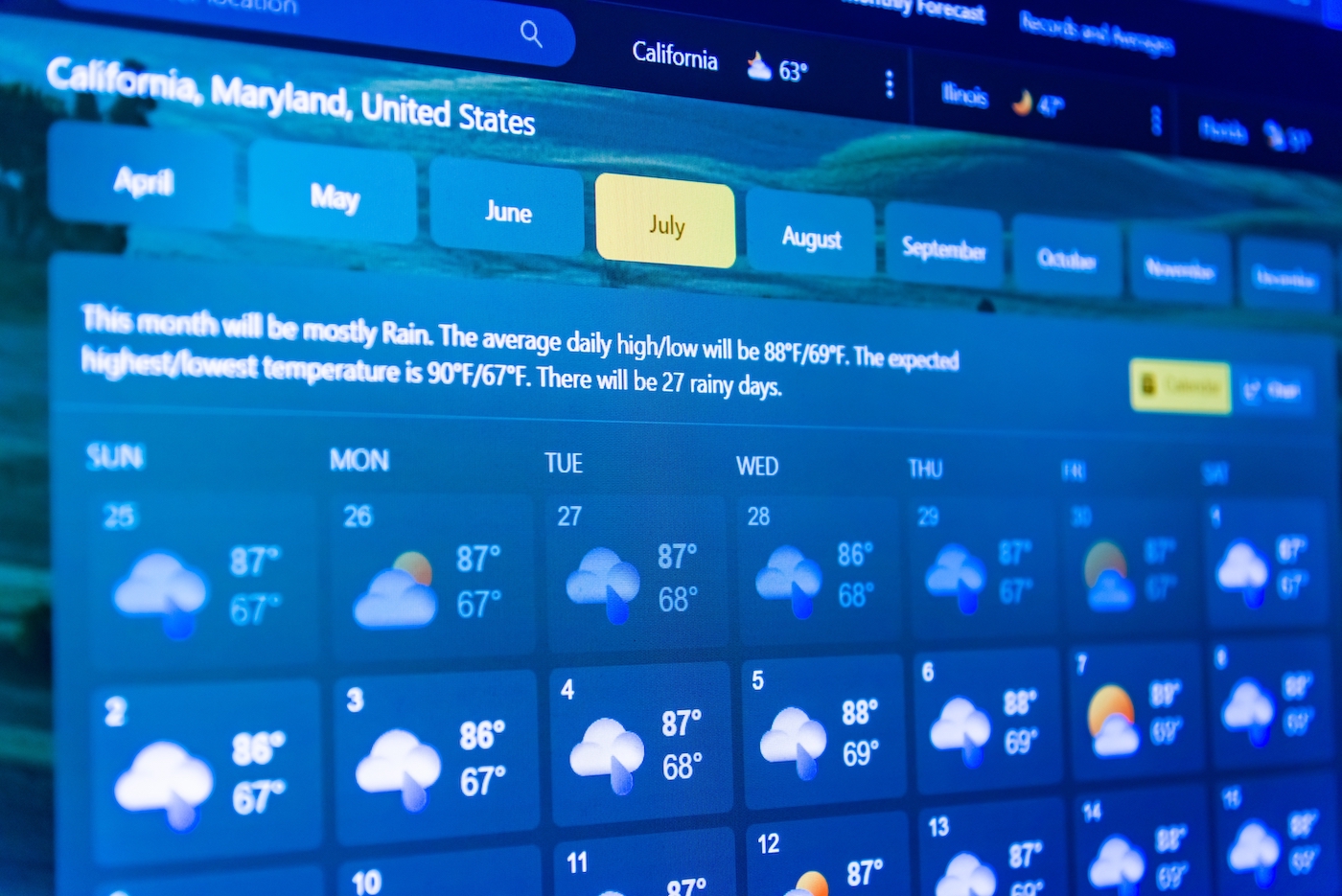
Access to weather forecasts has been made easier than ever with the advent of smartphones. Most of the time, we can get accurate information about weather for the next few hours up through the next few days. But a week or two out, those predictions get less reliable.
In the near future, it may be possible to get accurate weather forecasts weeks, months, or even a decade ahead of schedule. While this sounds like science fiction, researchers at the National Center for Atmospheric Research (NCAR) are working on this very challenge.
Earth system predictions, as the field is called, combines a variety of factors including atmospheric conditions, ocean currents, and even what’s happening in the soil to form predictions. These forecasts are in high demand as the climate changes, particularly as farmers need more information about incoming heat and precipitation. There’s even the possibility that Earth systems predictions could help regions prepare for dangerous natural hazards well ahead of time.
At Science Friday Live in Boulder, Colorado, Ira sat down with two NCAR scientists, Dr. Yaga Richter and Dr. Jerry Meehl about their work in this field.
Further Reading
- This radio segment was recorded in Boulder, Colorado at a live Science Friday event. To participate in one of our recordings in the future, check out our upcoming events page!
Sign Up For Our Events Newsletter
Join us for an upcoming SciFri Event—live, in-person, and online. Be the first to hear when we’ve got something new and exciting on the calendar by subscribing to our email newsletter.
Segment Transcript
The transcript of this segment is being processed. It will be available within one week after the show airs.
Meet the Producers and Host
About Ira Flatow
@iraflatowIra Flatow is the host and executive producer of Science Friday. His green thumb has revived many an office plant at death’s door.
About Kathleen Davis
@katjacquelineKathleen Davis is a producer at Science Friday, which means she spends the week brainstorming, researching, and writing, typically in that order. She’s a big fan of stories related to strange animal facts and dystopian technology.
About D. Peterschmidt
@dpeterschmidtD. Peterschmidt is a producer, host of the podcast Universe of Art, and composes music for Science Friday’s podcasts. Their D&D character is a clumsy bard named Chip Chap Chopman.